Evolution has achieved elegant solutions for problems that biological systems have to deal with. To me, the hallmark of these achievements is the complex, highly orchestrated and robust physiology of cells, when the underlying molecular events are basically random. For example, gene expression, a central process of the cell, is subject to significant noise due to thermal fluctuations and low copy numbers of biomolecules, such as DNA and mRNA. Despite this stochasticity, most cellular events are ordered and precisely regulated. Development in the worm C. elegans, for instance is so regular that we can trace the differentiated state of nearly every cell. Cells can even use noise to their benefit. Variation in protein numbers provides phenotypic diversity, maintaining each cells quantitative individuality.
I am broadly interested in understanding what enables cells to grow, divide, transmit signals, process information and make decisions in spite of the inherent stochasticity in the underlying biochemical networks. The goal of my research is to identify the principles that govern the design and robust function of networks of interacting genes and proteins. Such design principles are ones that have been consistently chosen by evolution.
Biological systems are too complex to be understood by studying their individual components alone. Systems-level study of biological networks demands a theoretical approach. Through the application of tools from physics, mathematics and bioinformatics, I study the temporal, spatial and stochastic dynamics of biochemical network models using analytical and computational methods. Experimental advances in high-throughput methods in molecular biology provide systems-level data, while single cell studies quantify variations in biomolecular levels and their corresponding phenotypic effects. Together, these data provide an excellent opportunity for system-level and quantitative studying of mechanism of robust cellular function and also phenotypic variability.
In my research, I strive to stay close to experimental data and we collaborate closely with biologists. My current collaborators include Omer Dushek (Oxford), Samuel Marguerat (MRC LMS), Dan Hebenstreit (Warwick), Brian Robertson (Imperial), Gerald Larrouy-Maumus (Imperial), Mark Isalan (Imperial), Peter Swain (Edinburgh).
In the following, I breifly describe some examples of recent work.
Multisite Phosphorylation and Switch-like Response
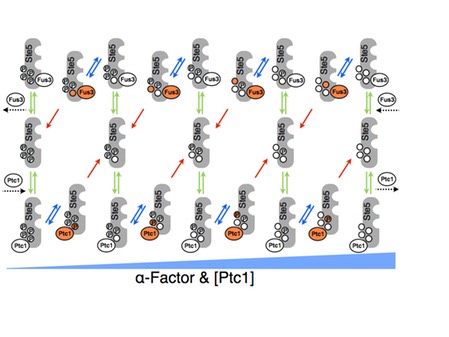

The haploid yeast Saccharomyces cerevisiae selects mating partners by interpreting the concentration gradient of pheromone secreted by potential mates through a network of mitogen activated protein kinase (MAPK) signalling proteins. The mating decision in yeast is an all-or-none, or switch-like, response that allows cells to filter weak pheromone signals, thus avoiding inappropriate commitment to mating by responding only at or above critical concentrations when a mate is sufficiently close. The molecular mechanisms that govern the switch-like mating decision are poorly understood. In collaboration with the lab of Stephen Michnick at the University of Montreal and Peter Swain at University of Edinburgh, we developed a model that explains the switch-like mating response (M. Malleshaiah, et al 2010 Nature 465, 101-105). Combining experiments and modeling, we showed that the switching mechanism arises from competition between the MAPK Fus3 and a phosphatase Ptc1 for control of the phosphorylation state of four sites on the scaffold protein Ste5. This competition results in a switch-like dissociation of Fus3 from Ste5 that is necessary to generate the switch-like mating response. Thus, the decision to mate is made at an early stage in the pheromone pathway and occurs rapidly, perhaps to prevent the loss of the potential mate to competitors. We argued that the architecture of the Fus3-Ste5-Ptc1 circuit generates a novel ultrasensitivity mechanism, which is robust to variations in the concentrations of these proteins. This robustness helps assure that mating can occur despite stochastic or genetic variation between individuals.
T cell receptors have about 20 phosphorylation sites. Motivated by yeast mating pathway model, and in collaboration with Omer Dushek at Oxford we recently looked at the effect of local saturation of enzymes due to slow membrane diffusion and multisite phosphorylation (O. Dushek, et al 2011 Biophys. J. 100, 1189-1197). Through spatial and stochastic simulations we have shown that multisite phosphorylation in membrane proteins can contribute to a sharp switch like response if we allow enzymatic inactivation in our model.
Stochastic Gene Expression and Intrinisic and Extrinsic Noise
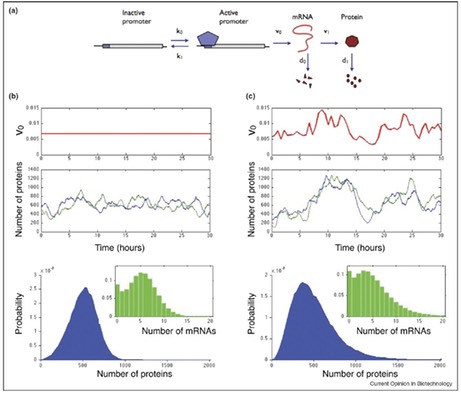
Although stochastic effects in biological networks have been studied extensively, most research has focused only on coefficient of variation and on intrinsic noise arising from the stochastic dynamics of the system. We presented an approximation that allows the calculation of not only the mean and variance, but also the distribution of protein numbers arising from intrinsic noise in gene expression (V Shahrezaei and PS Swain 2008 PNAS 105, 17256). To do this, we assumed that proteins decay substantially more slowly than their mRNA and conrmed that many genes satisfy this relation by using high-throughput data from budding yeast. Importantly, we found that protein distributions are in general asymmetric and may be poorly characterized only by their mean and variance.
Most studies of noise have neglected extrinsic fluctuations, which arise from the interactions of the system of interest with other stochastic systems in the cell or its environment. Yet, these fluctuations have been determined experimentally to generate most cellular variation. Such extrinsic fluctuations are non-specific, affecting many system components, and are colored, having a timescale comparable to the cell cycle. In order to include extrinsic as well as intrinsic fluctuations, we extended the standard stochastic simulation method (the Gillespie algorithm) to include time varying parameters. This enables inclusion of extrinsic fluctuations as well as intrinsic fluctua- tions. We showed that extrinsic fluctuations can profoundly affect the behavior of genetic networks: changing mean protein numbers, intrinsic noise, accelerating system response times, and combining constructively or destructively to amplify or attenuate system stochasticity (V Shahrezaei, et al, 2008 Mol Sys Biol 4, 196). In addition, these observations explained recent puzzling results from high throughput measurements of noise. Our method provides a general, dynamic and stochastic method for sensitivity analysis in biological systems and I believe it will become very useful for modeling biochemical networks in the presence of external fluctuations.